It has been widely recorded that the population neuronal activities pocess the low dimensional manifold in multiple brain regions, especially PFC. The origin of low dimensional dynamics and the relation between the dynamical properties and the network structures remain open questions. One of the potential solutions is that low dimensional dynamics is generated by low-rank network architectures. This work trained low-rank recurrent neural networks to perform 5 distinct cognitive tasks respectively, and theoretically analyzed the network dynamics performing computation for each task. Their work showed that very few ranks (1-2) of network structure are actually required to well perform those cognitive tasks. For those tasks with flexible input-target mapping, multiple cell-types (sub-populations) are necessary to perform tasks. Overall, their theory of low-rank RNN can extract the effective latent dynamics for computation, and furthermore provide a framework to networks with multitasking ability.
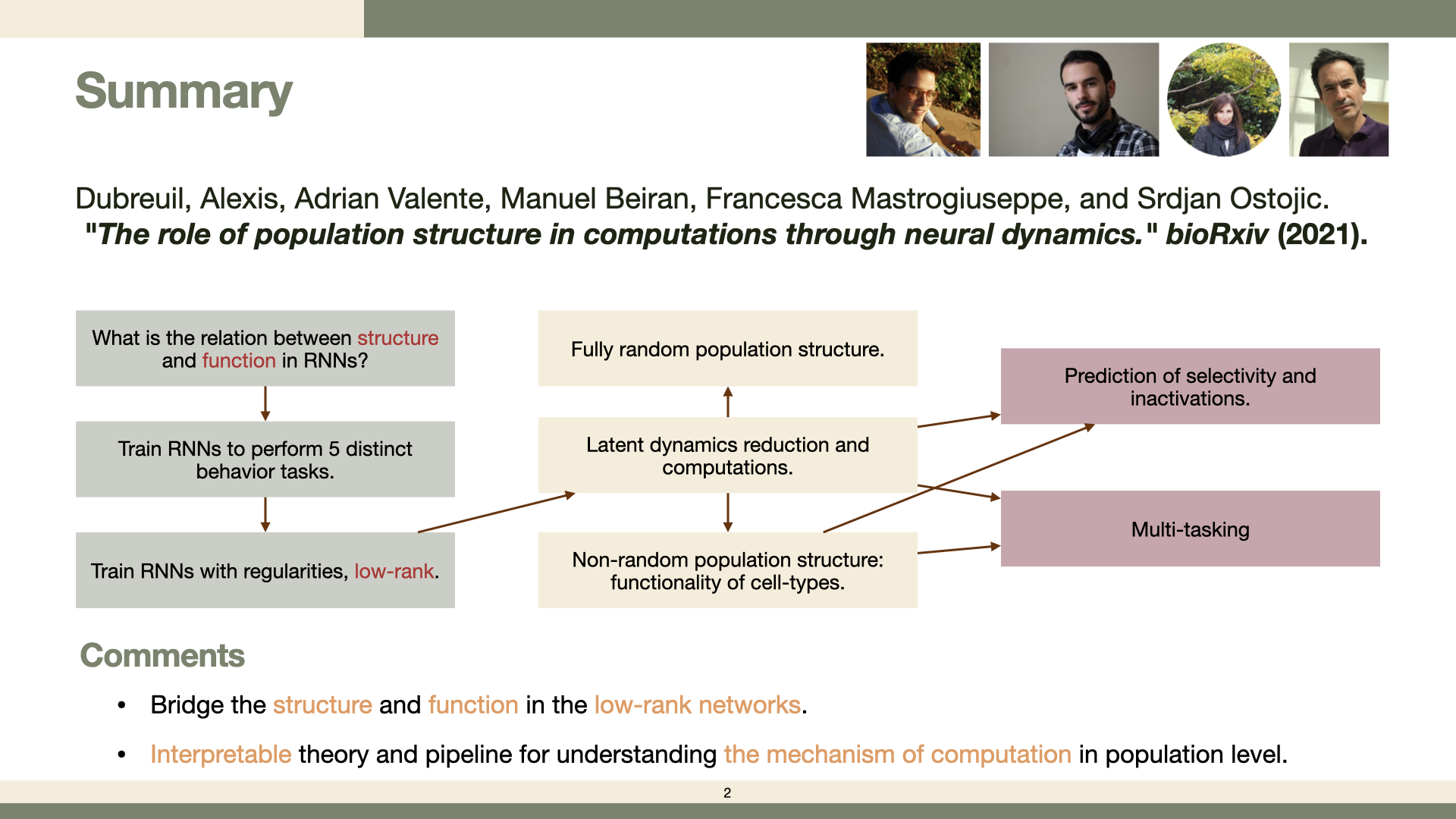